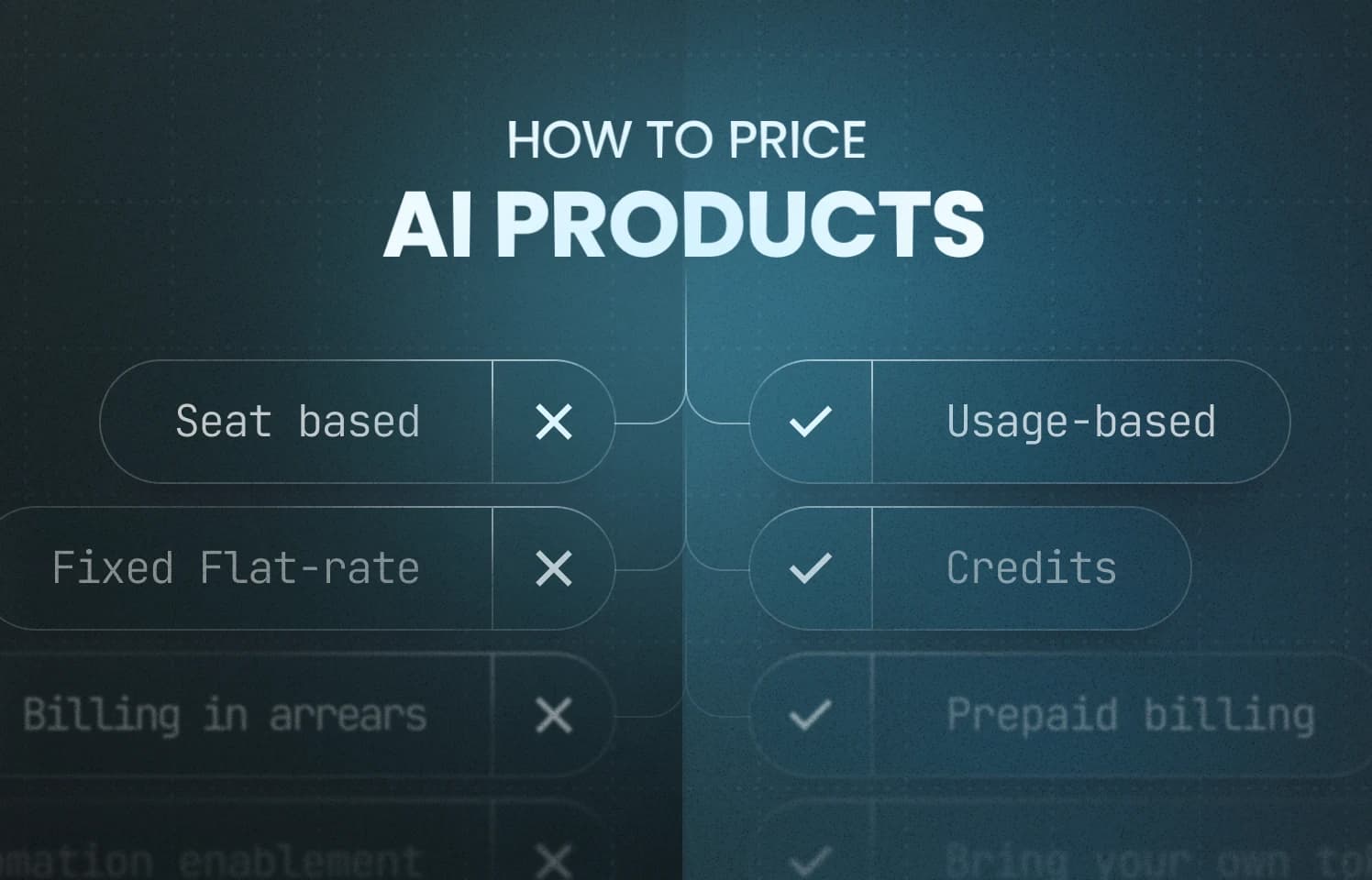
Pricing AI products poses unique challenges due to the high cost of goods and increased automation. We see AI companies experiment with multiple models, like usage-based pricing, adopting credits, and prepaid billing. This article will discuss AI-specific pricing challenges and explore models that can help overcome them.
The AI Pricing Challenge
At OpenMeter, we are helping emerging AI companies monetize their innovations. We are privileged to have insights into their pricing strategies and the thought processes that shape these decisions.
The biggest challenges in pricing AI products include:
- Rapidly growing product usage and significant automation demands
- Reliance on costly resources such as large language models (LLMs) and GPUs.
- Customers' desire for predictable and controllable spending
- High upfront costs to serve demand
Ineffective Models
Pricing models that don't protect margins or enable growth for automation are not a good fit for AI.
Seat-Based Pricing
Traditionally successful in enterprises, seat-based pricing doesn't fit AI well due to its high automation and limited early adopter engagement. In other words, most AI usage comes from a small number of power users and automation. Cost is another challenge here. This model often fails to ensure sustainable margins.
Plans With Fixed Flat-Rate
Flat-rate plans face challenges in AI monetization due to variable consumption rates and high underlying costs. AI companies often incorporate usage limits to protect margins or adopt hybrid pricing with usage-based components. We will talk about both concepts in the following sections.
Effective Pricing Strategies
Let's examine what pricing models and practices work for AI products. As always, no solution fits all, and most companies try out multiple models as they iterate on their pricing.
Usage-Based Pricing
In November 2022, OpenAI rolled out ChatGPT with a pure usage-based pricing model. Users paid after their token consumption. At the time, this pricing followed the billing-in-arrears model, where customers were billed after their consumption at the end of the month. Since then, in February 2024, OpenAI has changed from post-consumption billing to upfront prepaid payment.
OpenAI wasn't the first company to adopt usage-based billing. However, it was a significant event in AI pricing as it started to make consumers think about the number of tokens. Also, an increased influx of new AI startups, many building on foundational models like ChatGPT, started implementing cost-plus pricing to control their spending.
Cost Plus Pricing
As SaaS typically generates higher profit margins on value-based pricing, cost-plus pricing has been considered non-favorable in product and pricing circles. Many AI companies prefer cost-plus pricing as it aligns well with usage-based models, allowing companies to transparently manage costs and margins by marking up base costs from providers like OpenAI.
Bring Your Own API Key
An extreme variant of cost-plus pricing involves customers providing their OpenAI API keys, with companies only adding a platform fee. This model fully transfers foundational costs to the customer, allowing for transparent and direct pricing.
Success-Based Pricing
This approach charges customers based on the results delivered, such as the resolution of support tickets, rather than mere usage metrics. This model is viral in customer service AI implementations.
Expect to hear more about success-based pricing in the upcoming months as AI advances to resolve more complex tasks.
Hybrid Pricing Models
We see hybrid pricing gaining popularity when existing products providing flat-rate plans introduce new AI capabilities. A good example is Intercom, where you pay for Fin AI resolutions in addition to your traditional fixed-price plan. Another use case is when AI products with variadic consumption patterns want to ensure a baseline revenue. This is usually done with credits, where the customer gets a certain amount every month and can either buy more or pay overage fees.
Credits and Limits
Credits and limits play an increasing role in AI monetization as they can protect margins for flat-rate plans and ensure a baseline revenue for usage-based pricing models. We see multiple applications of credits and limits, sometimes in combination:
- Hard usage limits and quotes to protect margins with flat-rate plans
- Soft usage limits to drive expansion to larger plans
- Monthly recurring credit grants to ensure baseline revenue
- Prepaid credit top-ups to help customers control their spend
Credits can also cover upfront costs and minimize the number of payment transactions and fees for small accounts.
Prepaid Billing
Moving away from traditional post-consumption billing, AI vendors increasingly opt for prepaid solutions. This shift helps manage the upfront costs of providing AI services and mitigates customer concerns over unexpectedly high charges. In most cases, it combines credits, wallets, and tokens where customers can buy tokens upfront and top up their balance as they use more.
Summary
AI monetization is evolving before our eyes as AI takes over the world. AI companies continuously evaluate and adapt their pricing to balance cost, customer satisfaction, and competitive positioning. Usage-based pricing helps manage margins, while limits and prepaid credits can help both vendors and customers control their spending.
Do you need help with AI monetization?
OpenMeter can help to collect usage data, manage credits, and enforce limits.